AI governance
What is AI governance?
AI Governance is the set of frameworks, rules, and best practices to ensure responsible adoption and usage of artificial intelligence. This framework encourages organizations to curate and use bias free data, consider societal and end-user impact, and produce unbiased models; the framework also enforces controls on model progression through deployment stages. AI Governance is critical for organizations to realize the maximum value out of AI projects while mitigating risks. It enables organizations to not only develop AI projects in a responsible way, but also ensure that there is consistency and transparency across the entire organization.
H2O.ai's AI governance with H2O Model Validation
H2O.ai's AI governance framework recommends four stages and a total of 11 topics. Organizations are encouraged to adopt the topics and processes most relevant to their unique needs. The H2O.ai's AI governance framework is discussed in the following guide: Guidelines for Effective AI Governance with Applications in H2O AI Cloud.
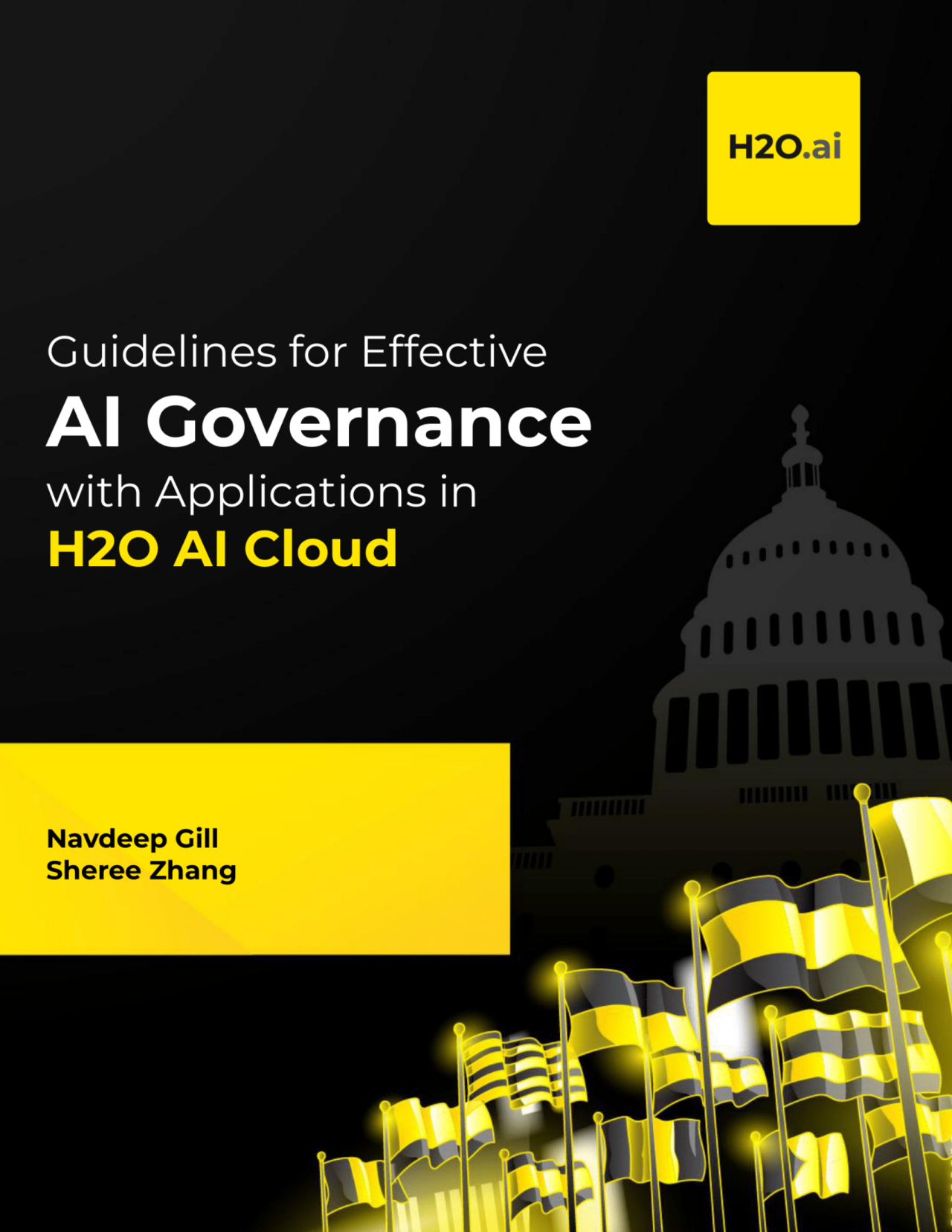
For example, based on the content of the guide, H2O Model Validation can help with AI governance in one of the following ways:
Prevent model degradation: H2O Model Validation can help with AI governance by addressing model degradation. H2O Model Validation provides various validation tests that can reveal weaknesses and vulnerabilities in datasets and models, thereby helping to prevent model degradation and enhance the overall governance of their AI systems.
Unintended biases or errors: H2O Model Validation's ability to assess the robustness and stability of trained models can help organizations ensure that their models perform as intended and do not introduce unintended biases or errors. By catching these issues early on, organizations can avoid potential negative consequences and maintain the integrity of their AI systems.
Incident response plan and escalation: H2O Model Validation can be used to assess the robustness and stability of trained models. This can help set up a robust response and escalation process as part of an AI governance framework, promoting a coordinated and timely response to issues arising from an ML system.
- Submit and view feedback for this page
- Send feedback about H2O Model Validation to cloud-feedback@h2o.ai