Deploying the MOJO Pipeline¶
Driverless AI can deploy the MOJO scoring pipeline for you to test and/or to integrate into a final product.
Notes:
- This section describes how to deploy a MOJO scoring pipeline and assumes that a MOJO scoring pipeline exists. Refer to the Driverless AI MOJO Scoring Pipeline section for information on how to build a MOJO scoring pipeline.
- This is an early feature that will eventually support multiple different deployments. At this point, Driverless AI can only deploy the trained MOJO scoring pipeline as an AWS Lambda Function, i.e., a server-less scorer running in Amazon Cloud and charged by the actual usage.
Deployments Overview Page¶
All of the MOJO scoring pipeline deployments are available in the Deployments Overview page, which is available from the top menu. This page lists all active deployments and the information needed to access the respective endpoints. In addition, it allows you to stop any deployments that are no longer needed.

Amazon Lambda Deployment¶
Driverless AI Prerequisites¶
- Driverless AI MOJO Scoring Pipeline: To deploy a MOJO scoring pipeline as an AWS lambda function, the MOJO pipeline archive has to be created first by choosing the Build MOJO Scoring Pipeline option on the completed experiment page. Refer to the Driverless AI MOJO Scoring Pipeline section for information on how to build a MOJO scoring pipeline.
- Terraform v0.11.x (specifically v0.11.10 or greater): In addition, the
Terrafrom
tool (https://www.terraform.io/) has to be installed on the system running Driverless AI. The tool is included in the Driverless AI Docker images but not in native install packages. To install Terraform, please follow the steps on Terraform installation page.
Notes:
- Terraform is not available on every platform. In particular, there is no Power build, so AWS Lambda Deployment is currently not supported on Power installations of Driverless AI.
- Terraform v0.12 is not supported. If you have v0.12 installed, you will need to download to v0.11.x (specifically v0.11.10 or greater) in order to deploy a MOJO scoring pipeline as an AWS lambda function.
AWS Prerequisites¶
Usage Plans¶
Usage plans must be enabled in the target AWS region in order for API keys to work when accessing the AWS Lambda via its REST API. Refer to https://aws.amazon.com/blogs/aws/new-usage-plans-for-amazon-api-gateway/ for more information.
Access Permissions¶
The following AWS access permissions need to be provided to the role in order for Driverless AI Lambda deployment to succeed.
- AWSLambdaFullAccess
- IAMFullAccess
- AmazonAPIGatewayAdministrator
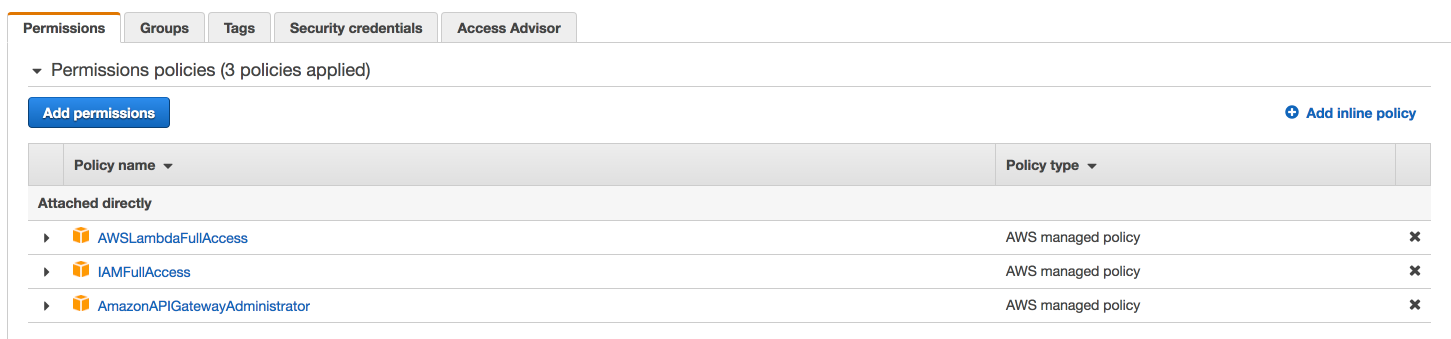
The policy can be further stripped down to restrict Lambda and S3 rights using the JSON policy definition as follows:
{
"Version": "2012-10-17",
"Statement": [
{
"Sid": "VisualEditor0",
"Effect": "Allow",
"Action": [
"iam:GetPolicyVersion",
"iam:DeletePolicy",
"iam:CreateRole",
"iam:AttachRolePolicy",
"iam:ListInstanceProfilesForRole",
"iam:PassRole",
"iam:DetachRolePolicy",
"iam:ListAttachedRolePolicies",
"iam:GetRole",
"iam:GetPolicy",
"iam:DeleteRole",
"iam:CreatePolicy",
"iam:ListPolicyVersions"
],
"Resource": [
"arn:aws:iam::*:role/h2oai*",
"arn:aws:iam::*:policy/h2oai*"
]
},
{
"Sid": "VisualEditor1",
"Effect": "Allow",
"Action": "apigateway:*",
"Resource": "*"
},
{
"Sid": "VisualEditor2",
"Effect": "Allow",
"Action": [
"lambda:CreateFunction",
"lambda:ListFunctions",
"lambda:InvokeFunction",
"lambda:GetFunction",
"lambda:UpdateFunctionConfiguration",
"lambda:DeleteFunctionConcurrency",
"lambda:RemovePermission",
"lambda:UpdateFunctionCode",
"lambda:AddPermission",
"lambda:ListVersionsByFunction",
"lambda:GetFunctionConfiguration",
"lambda:DeleteFunction",
"lambda:PutFunctionConcurrency",
"lambda:GetPolicy"
],
"Resource": "arn:aws:lambda:*:*:function:h2oai*"
},
{
"Sid": "VisualEditor3",
"Effect": "Allow",
"Action": "s3:*",
"Resource": [
"arn:aws:s3:::h2oai*/*",
"arn:aws:s3:::h2oai*"
]
}
]
}
Deploying on Amazon Lambda¶
Once the MOJO pipeline archive is ready, Driverless AI provides a Deploy option on the completed experiment page.
Notes:
- This button is only available after the MOJO Scoring Pipeline has been built.
- This button is not available on PPC64LE environments.
This option opens a new dialog for setting the AWS account credentials (or use those supplied in the Driverless AI configuration file or environment variables), AWS region, and the desired deployment name (which must be unique per Driverless AI user and AWS account used).
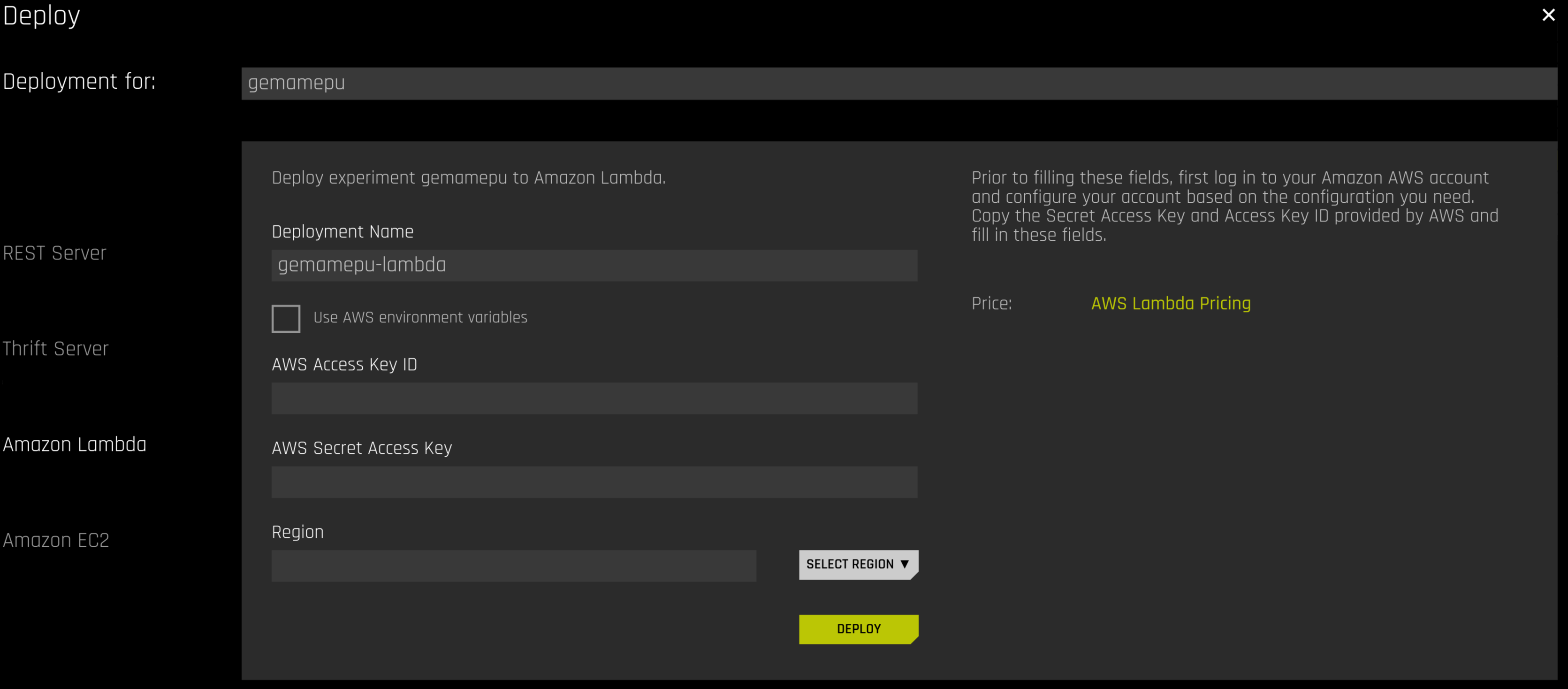
Amazon Lambda deployment parameters:
- Deployment Name: A unique name of the deployment. By default, Driverless AI offers a name based on the name of the experiment and the deployment type. This has to be unique both for Driverless AI user and the AWS account used.
- Region: The AWS region to deploy the MOJO scoring pipeline to. It makes sense to choose a region geographically close to any client code calling the endpoint in order to minimize request latency. (See also AWS Regions and Availability Zones.)
- Use AWS environment variables: If enabled, the AWS credentials are taken from the Driverless AI configuration file (see records
deployment_aws_access_key_id
anddeployment_aws_secret_access_key
) or environment variables (DRIVERLESS_AI_DEPLOYMENT_AWS_ACCESS_KEY_ID
andDRIVERLESS_AI_DEPLOYMENT_AWS_SECRET_ACCESS_KEY
). This would usually be entered by the Driverless AI installation administrator.- AWS Access Key ID and AWS Secret Access Key: Credentials to access the AWS account. This pair of secrets identifies the AWS user and the account and can be obtained from the AWS account console.
Testing the Lambda Deployment¶
On a successful deployment, all the information needed to access the new endpoint (URL and an API Key) is printed, and the same information is available in the Deployments Overview Page after clicking on the deployment row.
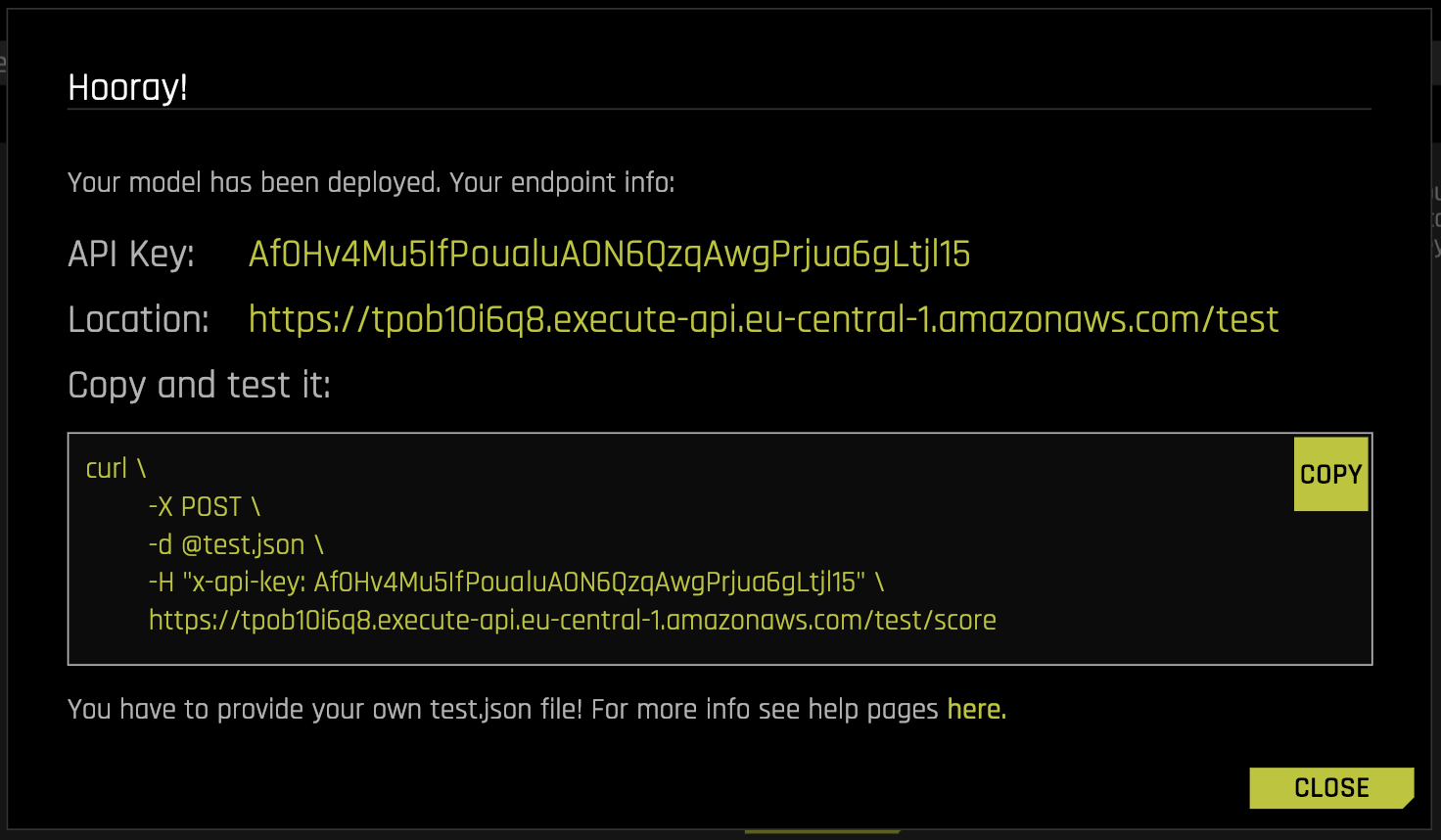
Note that the actual scoring endpoint is located at the path /score
. In addition, to prevent DDoS and other malicious activities, the resulting AWS lambda is protected by an API Key, i.e., a secret that has to be passed in as a part of the request using the x-api-key
HTTP header.
The request is a JSON object containing attributes:
- fields: A list of input column names that should correspond to the training data columns.
- rows: A list of rows that are in turn lists of cell values to predict the target values for.
- optional includeFieldsInOutput: A list of input columns that should be included in the output.
An example request providing 2 columns on the input and asking to get one column copied to the output looks as follows:
{
"fields": [
"age", "salary"
],
"includeFieldsInOutput": [
"salary"
],
"rows": [
[
"48.0", "15000.0"
],
[
"35.0", "35000.0"
],
[
"18.0", "22000.0"
]
]
}
Assuming the request is stored locally in a file named test.json
, the request to the endpoint can be sent, e.g., using the curl
utility, as follows:
$ URL={place the endpoint URL here}
$ API_KEY={place the endpoint API key here}
$ curl \
-d @test.json \
-X POST \
-H "x-api-key: ${API_KEY}" \
${URL}/score
The response is a JSON object with a single attribute score
, which contains the list of rows with the optional copied input values and the predictions.
For the example above with a two class target field, the result is likely to look something like the following snippet. The particular values would of course depend on the scoring pipeline:
{
"score": [
[
"48.0",
"0.6240277982943945",
"0.045458571508101536",
],
[
"35.0",
"0.7209441819603676",
"0.06299909138586585",
],
[
"18.0",
"0.7209441819603676",
"0.06299909138586585",
]
]
}
AWS Deployment Issues¶
We create a new S3 bucket per AWS Lambda deployment. The bucket names have to be unique throughout AWS S3, and one user can create a maximum of 100 buckets. Therefore, we recommend setting the bucket name used for deployment with the deployment_aws_bucket_name
config option.