FAQ
H2O Driverless AI is an artificial intelligence (AI) platform for automatic machine learning. Driverless AI automates some of the most difficult data science and machine learning workflows such as feature engineering, model validation, model tuning, model selection and model deployment. It aims to achieve highest predictive accuracy, comparable to expert data scientists, but in much shorter time thanks to end-to-end automation. Driverless AI also offers automatic visualizations and machine learning interpretability (MLI). Especially in regulated industries, model transparency and explanation are just as important as predictive performance. Modeling pipelines (feature engineering and models) are exported (in full fidelity, without approximations) both as Python modules and as Java standalone scoring artifacts.
This section provides answers to frequently asked questions. If you have additional questions about using Driverless AI, post them on Stack Overflow using the driverless-ai tag at http://stackoverflow.com/questions/tagged/driverless-ai. You can also post questions on the H2O.ai Community Slack workspace in the #driverlessai channel. If you have not signed up for the H2O.ai Community Slack workspace, you can do so here: https://www.h2o.ai/community/.
General
How is Driverless AI different than any other black box ML algorithm?
How often do new versions come out?
Installation/Upgrade/Authentication
How can I change my username and password?
Can Driverless AI run on CPU-only machines?
How can I upgrade to a newer version of Driverless AI?
What kind of authentication is supported in Driverless AI?
How can I automatically turn on persistence each time the GPU system reboots?
How can I start Driverless AI on a different port than 12345?
Can I set up TLS/SSL on Driverless AI?
Can I set up TLS/SSL on Driverless AI in AWS?
Why do I receive a “package dai-<version>.x86_64 does not verify: no digest” error during the installation?
I received a “Must have exactly one OpenCL platform ‘NVIDIA CUDA’” error. How can I fix that?
Is it possible for multiple users to share a single Driverless AI instance?
Can multiple Driverless AI users share a GPU server?
How can I retrieve a list of Driverless AI users?
Start of Driverless AI fails on the message “Segmentation fault (core dumped)” on Ubuntu 18/RHEL 7.6. How can I fix this?
Which Linux systems does Driverless AI support?
Data
Is there a file size limit for datasets?
How can I import CSV files that use UTF-8 encoding into Excel?
Can a byte order mark be used when writing CSV files with datatable?
Which version of Longhorn is supported by Driverless AI?
Is it possible to download a transformed test dataset in Driverless AI?
How does DAI support small data?
Connectors
Why can’t I import a folder as a file when using a data connector on Windows?
I get a ClassNotFoundException error when I try to select a JDBC connection. How can I fix that?
I get a org.datanucleus.exceptions.NucleusUserException: Please check your CLASSPATH and plugin specification error when attempting to connect to hive. How can I fix that?
I get a “Permission Denied” error during Hive import. How do I fix this?
Recipes
Where can I retrieve H2O’s custom recipes?
How can I create my own custom recipe?
Are MOJOs supported for experiments that use custom recipes?
How can I use BYOR in my airgapped installation?
When enabling recipes in Driverless AI, can I install Python packages from my organization’s internal Python package index?
Experiments
How much memory does Driverless AI require in order to run experiments?
How many columns can Driverless AI handle?
How should I use Driverless AI if I have large data?
How does Driverless AI detect the ID column?
Can Driverless AI handle data with missing values/nulls?
How does Driverless AI deal with categorical variables? What if an integer column should really be treated as categorical?
How are outliers handled?
If I drop several columns from the Train dataset, will Driverless AI understand that it needs to drop the same columns from the Test dataset?
Does Driverless AI treat numeric variables as categorical variables?
Which algorithms are used in Driverless AI?
Why do my selected algorithms not show up in the Experiment Preview?
How can we turn on TensorFlow Neural Networks so they are evaluated?
Does Driverless AI standardize the data?
What objective function is used in XGBoost?
Does Driverless AI perform internal or external validation?
How does Driverless AI prevent overfitting?
How does Driverless AI avoid the multiple hypothesis (MH) problem?
How does Driverless AI suggest the experiment settings?
What happens when I set Interpretability and Accuracy to the same number?
Can I specify the number of GPUs to use when running Driverless AI?
How can I create the simplest model in Driverless AI?
Why is my experiment suddenly slow?
When I run multiple experiments with different seeds, why do I see different scores, runtimes, and sizes on disk in the Experiments listing page?
Why does the final model performance appear to be worse than previous iterations?
How can I find features that may be causing data leakages in my Driverless AI model?
How can I see the performance metrics on the test data?
How can I see all the performance metrics possible for my experiment?
What if my training/validation and testing data sets come from different distributions?
Does Driverless AI handle weighted data?
How does Driverless AI handle fold assignments for weighted data?
Why do I see that adding new features to a dataset deteriorates the performance of the model?
How does Driverless AI handle imbalanced data for binary classification experiments?
How is feature importance calculated in Driverless AI?
I want to have only one LightGBM model in the final pipeline. How can I achieve this?
I want to have only one LightGBM model and no FE. How can I do this?
What is fast approximation in Driverless AI?
When should fast approximation be turned off?
Why does the confusion matrix sometimes show decimals instead of whole numbers?
Is data sampling for multiclass use cases supported?
Feature Transformations
Where can I get details of the various transformations performed in an experiment?
Predictions
How can I download the predictions onto the machine where Driverless AI is running?
Why are predicted probabilities not available when I run an experiment without ensembling?
Deployment
What drives the size of a MOJO?
Are MOJOs thread safe?
Running the scoring pipeline for my MOJO is taking several hours. How can I get this to run faster?
Why have I encountered a “Best Score is not finite” error?
Time Series
What if my data has a time dependency?
What is a lag, and why does it help?
Why can’t I specify a validation data set for time-series problems? Why do you look at the test set for time-series problems
Why does the gap between train and test matter? Is it because of creating the lag features on the test set?
In regards to applying the target lags to different subsets of the time group columns, are you saying Driverless AI perform auto-correlation at “levels” of the time series? For example, consider the Walmart dataset where I have Store and Dept (and my target is Weekly Sales). Are you saying that Driverless AI checks for auto-correlation in Weekly Sales based on just Store, just Dept, and both Store and Dept?
How does Driverless AI detect the time period?
What is the logic behind the selectable numbers for forecast horizon length?
Assume that in my Walmart dataset, all stores provided data at the week level, but one store provided data at the day level. What would Driverless AI do?
Assume that in my Walmart dataset, all stores and departments provided data at the weekly level, but one department in a specific store provided weekly sales on a bi-weekly basis (every two weeks). What would Driverless AI do?
Why does the number of weeks that you want to start predicting matter?
Are the scoring components of time series sensitive to the order in which new pieces of data arrive? I.e., is each row independent at scoring time, or is there a real-time windowing effect in the scoring pieces?
What happens if the user, at predict time, gives a row with a time value that is too small or too large?
What’s the minimum data size for a time series recipe?
How long must the training data be compared to the test data?
How does the time series recipe deal with missing values?
Can the time information be distributed across multiple columns in the input data (such as [year, day, month]?
What type of modeling approach does Driverless AI use for time series?
What’s the idea behind exponential weighting of moving averages?
Logging
How can I reduce the size of the Audit Logger?
General
How is Driverless AI different than any other black box ML algorithm?
Driverless AI uses many techniques (some older and some cutting-edge) for interpreting black box models including creating reason codes for every prediction the system makes. We have also created numerous open source code examples and free publications that explain these techniques. See the list below for links to these resources and for references for the interpretability techniques.
Open source interpretability examples:
Free Machine Learning Interpretability publications:
Machine Learning Techniques already in Driverless AI:
Tree-based Variable Importance: https://web.stanford.edu/~hastie/ElemStatLearn/printings/ESLII_print12.pdf
Partial Dependence: https://web.stanford.edu/~hastie/ElemStatLearn/printings/ESLII_print12.pdf
LIME: http://www.kdd.org/kdd2016/papers/files/rfp0573-ribeiroA.pdf
LOCO: http://www.stat.cmu.edu/~ryantibs/papers/conformal.pdf
Surrogate Models:
Shapley Explanations: http://papers.nips.cc/paper/7062-a-unified-approach-to-interpreting-model-predictions
How often do new versions come out?
The frequency of major new Driverless AI releases has historically been about every two months.
Installation/Upgrade/Authentication
How can I change my username and password?
The username and password are tied to the experiments you have created. For example, if I log in with the username/password: megan/megan and start an experiment, then I would need to log back in with the same username and password to see those experiments. The username and password, however, does not limit your access to Driverless AI. If you want to use a new user name and password, you can log in again with a new username and password, but keep in mind that you won’t see your old experiments.
Can Driverless AI run on CPU-only machines?
Yes, Driverless AI can run on machines with CPUs only, though GPUs are recommended. Installation instructions are available for GPU and CPU systems. Refer to Before You Begin for more information.
How can I upgrade to a newer version of Driverless AI?
Upgrade instructions vary depending on your environment. Refer to the installation section for your environment. Upgrade instructions are included there.
What kind of authentication is supported in Driverless AI?
Driverless AI supports Client Certificate, LDAP, Local, mTLS, OpenID, none, and unvalidated (default) authentication. These can be configured by setting the appropriate environment variables in the config.toml file or by specifying the environment variables when starting Driverless AI. Refer to Authentication Methods for more information.
How can I automatically turn on persistence each time the GPU system reboots?
For GPU machines, the
sudo nvidia-persistenced --user dai
command can be run after each reboot to enable persistence. For systems that have systemd, it is possible to automatically enable persistence after each reboot by removing the--no-persistence-mode
flag from nvidia-persistenced.service. Before running the steps below, be sure to review the following for more information:
https://docs.nvidia.com/deploy/driver-persistence/index.html#persistence-daemon
https://docs.nvidia.com/deploy/driver-persistence/index.html#installation
Run the following to stop the nvidia-persistenced.service:
sudo systemctl stop nvidia-persistenced.service
Open the file /lib/systemd/system/nvidia-persistenced.service. This file includes a line “ExecStart=/usr/bin/nvidia-persistenced –user nvidia-persistenced –no-persistence-mode –verbose”.
Remove the flag
--no-persistence-mode
from that line so that it reads:ExecStart=/usr/bin/nvidia-persistenced --user nvidia-persistenced --verbose
Run the following command to start the nvidia-persistenced.service:
sudo systemctl start nvidia-persistenced.service
How can I start Driverless AI on a different port than 12345?
When starting Driverless AI in Docker, the
-p
option specifies the port on which Driverless AI will run. Change this option in the start script if you need to run on a port other than 12345. The following example shows how to run on port 22345. (Changenvidia-docker run
todocker-run
if needed.) Keep in mind that priviliged ports will require root access.nvidia-docker run \ --pid=host \ --init \ --rm \ --shm-size=2g --cap-add=SYS_NICE --ulimit nofile=131071:131071 --ulimit nproc=16384:16384 \ -u `id -u`:`id -g` \ -p 22345:12345 \ -v `pwd`/data:/data \ -v `pwd`/log:/log \ -v `pwd`/license:/license \ -v `pwd`/tmp:/tmp \ h2oai/dai-ubi8-x86_64:2.0.0-cuda11.8.0.xxTo run on a port other than 12345, update the port value in the config.toml file. The following example shows how to run Driverless AI on port 22345. Keep in mind that priviliged ports will require root access.
# Export the Driverless AI config.toml file (or add it to ~/.bashrc) export DRIVERLESS_AI_CONFIG_FILE=“/config/config.toml” # IP address and port for Driverless AI HTTP server. ip = "127.0.0.1" port = 22345Point to this updated config file when restarting Driverless AI.
Can I set up TLS/SSL on Driverless AI?
Yes, Driverless AI provides configuration options that let you set up HTTPS/TLS/SSL. You will need to have your own SSL certificate, or you can create a self-signed certificate for yourself.
To enable HTTPS/TLS/SSL on the Driverless AI server, add the following to the config.toml file:
enable_https = true ssl_key_file = "/etc/dai/private_key.pem" ssl_crt_file = "/etc/dai/cert.pem"You can make a self-signed certificate for testing with the following commands:
umask 077 openssl req -x509 -newkey rsa:4096 -keyout private_key.pem -out cert.pem -days 20 -nodes -subj '/O=Driverless AI' sudo chown dai:dai cert.pem private_key.pem sudo mv cert.pem private_key.pem /etc/daiTo configure specific versions of TLS/SSL, enable or disable the following settings in the config.toml file:
ssl_no_sslv2 = true ssl_no_sslv3 = true ssl_no_tlsv1 = true ssl_no_tlsv1_1 = true ssl_no_tlsv1_2 = false ssl_no_tlsv1_3 = false
Can I set up TLS/SSL on Driverless AI in AWS?
Yes, you can set up HTTPS/TLS/SSL on Driverless AI running in an AWS environment. HTTPS/TLS/SSL needs to be configured on the host machine, and the necessary ports will need to be opened on the AWS side. You will need to have your own TLS/SSL cert or you can create a self signed cert for yourself.
The following is a very simple example showing how to configure HTTPS with a proxy pass to the port on the container 12345 with the keys placed in /etc/nginx/. Replace <server_name> with your server name.
server { listen 80; return 301 https://$host$request_uri; } server { listen 443; # Specify your server name here server_name <server_name>; ssl_certificate /etc/nginx/cert.crt; ssl_certificate_key /etc/nginx/cert.key; ssl on; ssl_session_cache builtin:1000 shared:SSL:10m; ssl_protocols TLSv1 TLSv1.1 TLSv1.2; ssl_ciphers HIGH:!aNULL:!eNULL:!EXPORT:!CAMELLIA:!DES:!MD5:!PSK:!RC4; ssl_prefer_server_ciphers on; access_log /var/log/nginx/dai.access.log; location / { proxy_set_header Host $host; proxy_set_header X-Real-IP $remote_addr; proxy_set_header X-Forwarded-For $proxy_add_x_forwarded_for; proxy_set_header X-Forwarded-Proto $scheme; # Fix the “It appears that your reverse proxy set up is broken" error. proxy_pass http://localhost:12345; proxy_read_timeout 90; # Specify your server name for the redirect proxy_redirect http://localhost:12345 https://<server_name>; } }More information about SSL for Nginx in Ubuntu 16.04 can be found here: https://www.digitalocean.com/community/tutorials/how-to-create-a-self-signed-ssl-certificate-for-nginx-in-ubuntu-16-04.
I received a “package dai-<version>.x86_64 does not verify: no digest” error during the installation. How can I fix this?
You will recieve a “package dai-<version>.x86_64 does not verify: no digest” error when installing the rpm using an RPM version newer than 4.11.3. You can run the following as a workaround, replacing
<version>
with your DAI version:rpm --nodigest -i dai-<version>.x86_64.rpm
I received a “Must have exactly one OpenCL platform ‘NVIDIA CUDA’” error. How can I fix that?
If you encounter problems with opencl errors at server time, you may see the following message:
2018-11-08 14:26:15,341 C: D:452.2GB M:246.0GB 21603 ERROR : Must have exactly one OpenCL platform 'NVIDIA CUDA', but got: Platform #0: Clover Platform #1: NVIDIA CUDA +-- Device #0: GeForce GTX 1080 Ti +-- Device #1: GeForce GTX 1080 Ti +-- Device #2: GeForce GTX 1080 Ti Uninstall all but 'NVIDIA CUDA' platform.For Ubuntu, the solution is to run the following:
sudo apt-get remove mesa-opencl-icd
Is it possible for multiple users to share a single Driverless AI instance?
Driverless AI supports multiple users, and Driverless AI is licensed per a single named user. Therefore, in order, to have different users run experiments simultaneously, they would each need a license. Driverless AI manages the GPU(s) that it is given and ensures that different experiments from different users can run safely simultaneously and don’t interfere with each other. So when two licensed users log in with different credentials, then neither of them will see the other’s experiment. Similarly, if a licensed user logs in using a different set of credentials, then that user will not see any previously run experiments.
Yes, you can allocate multiple users in a single GPU box. For example, a single box with four GPUs can allocate that User1 has two GPUs and User2 has the other two GPUs. This is accomplished by having two separated Driverless AI instances running on the same server.
There are two ways to assign specific GPUs to Driverless AI. And in the scenario with four GPUs (two GPUs allocated to two users), both of these options allow each Docker container only to see two GPUs.
Use the
CUDA_VISIBLE_DEVICES
environment variable. In the case of Docker deployment, this will translate in passing the-e CUDA_VISIBLE_DEVICES="0,1"
to thenvidia-docker run
command.Passing the
NV_GPU
option at the beginning of thenvidia-docker run
command. (See example below.)#Team 1 NV_GPU='0,1' nvidia-docker run --pid=host --init --rm --shm-size=2g --cap-add=SYS_NICE --ulimit nofile=131071:131071 --ulimit nproc=16384:16384 -u id -u:id -g -p port-to-team:12345 -e DRIVERLESS_AI_CONFIG_FILE="/config/config.toml" -v /data:/data -v /log:/log -v /license:/license -v /tmp:/tmp -v /config:/config h2oai/dai-ubi8-x86_64:2.0.0-cuda11.8.0.xx #Team 2 NV_GPU='0,1' nvidia-docker run --pid=host --init --rm --shm-size=2g --cap-add=SYS_NICE --ulimit nofile=131071:131071 --ulimit nproc=16384:16384 -u id -u:id -g -p port-to-team:12345 -e DRIVERLESS_AI_CONFIG_FILE="/config/config.toml" -v /data:/data -v /log:/log -v /license:/license -v /tmp:/tmp -v /config:/config h2oai/dai-ubi8-x86_64:2.0.0-cuda11.8.0.xxNote, however, that a Driverless AI instance expects to fully utilize and not share the GPUs that are assigned to it. Sharing a GPU with other Driverless AI instances or other running programs can result in out-of-memory issues.
How can I retrieve a list of Driverless AI users?
A list of users can be retrieved using the Python client.
h2o = Client(address='http://<client_url>:12345', username='<username>', password='<password>') h2o.get_users()
Start of Driverless AI fails on the message ``Segmentation fault (core dumped)`` on Ubuntu 18/RHEL 7.6. How can I fix this?
This problem is caused by the font
NotoColorEmoji.ttf
, which cannot be processed by the Python matplotlib library. A workaround is to disable the font by renaming it. (Do not use fontconfig because it is ignored by matplotlib.) The following will print out the command that should be executed.sudo find / -name "NotoColorEmoji.ttf" 2>/dev/null | xargs -I{} echo sudo mv {} {}.backup
Which Linux systems does Driverless AI support?
Supported Linux systems include x86_64 RHEL 7, RHEL 8, CentOS 7, and CentOS 8.
Data
Is there a file size limit for datasets?
For GBMs, the file size for datasets is limited by the collective CPU or GPU memory on the system, but we continue to make optimizations for getting more data into an experiment, such as using TensorFlow streaming to stream to arbitrarily large datasets.
How can I import CSV files that use UTF-8 encoding into Excel?
Excel requires a byte order mark (BOM) to correctly identify CSV files that use UTF-8 encoding. Refer to the following FAQ entry for more information on how to use a BOM when writing CSV files with datatable.
Can a byte order mark be used when writing CSV files with datatable?
Yes, a byte order mark (BOM) can be used when writing CSV files with datatable by enabling
datatable_bom_csv
in the config.toml file when starting Driverless AI.Note: Support for UTF-8 encoding in Excel requires the use of a BOM.
Which version of Longhorn is supported by Driverless AI?
Driverless AI supports Longhorn v1.1.0 or later.
Is it possible to download a transformed test dataset in Driverless AI?
Yes, a transformed test dataset can be downloaded in Driverless AI. To do this, click Model Actions > Transform Dataset on the completed experiment page, then specify both a train and a test dataset to use for the transformation. The transformed test dataset is made available for download once this process is completed.
How does DAI support small data?
H2O Driverless AI automatically suggests experiment settings based on the number of columns and number of rows in your dataset. The settings are suggested to ensure best handling when the data is small. If the data is small, Driverless AI automatically suggests the experiment settings that prevent overfitting and ensure that the full dataset is utilized:
If the number of rows and number of columns are each below a certain threshold, then Accuracy is increased to 8 so that cross validation is performed. (We don’t want to “throw away” any data for internal validation purposes.)
Interpretability is increased up to 8. The higher the interpretability setting, the smaller the number of features in the final model. More complex features are not allowed. This prevents overfitting.
Time will be decreased down to 2. This ensures that there will be fewer feature engineering iterations, and thus prevents overfitting.
In addition to the preceding experiment setting suggestions, DAI also improves small data handling by:
Only target encoding features with strong signal.
Speed up BertModel and BertTransformer when data is text-dominated to avoid unnecessary validation repeats for small data.
Repeated cross-validation for final ensembles for small data, resulting in improved accuracy.
Always perform stratified sampling for binary classification problems, even if the imbalance ratio is less than 1:100. This can improve model robustness for small data.
Efficient handling of small data by running more tasks in parallel with single core to more efficiently use all cores.
Connectors
Why can’t I import a folder as a file when using a data connector on Windows?
If you try to use the Import Folder as File option via a data connector on Windows, the import will fail if the folder contains files that do not have file extensions. For example, if a folder contains the files file1.csv, file2.csv, file3.csv, and _SUCCESS, the function will fail due to the presence of the _SUCCESS file.
Note that this only occurs if the data is sourced from a volume that is mounted from the Windows filesystem onto the Docker container via
-v /path/to/windows/filesystem:/path/in/docker/container
flags. This error occurs because of the difference in how files without file extensions are treated in Windows and in the Docker container (CentOS Linux).
I get a ClassNotFoundException error when I try to select a JDBC connection. How can I fix that?
The folder storing the JDBC jar file must be visible/readable by the dai process user.
If you downloaded the JDBC jar file from Oracle, they may provide you with a tar.gz file that you can unpackage with the following command:
tar --no-same-permissions --no-same-owner -xzvf <my-jdbc-driver.tar>.gzAlternatively you can ensure that the permissions on the file are correct in general by running the following:
chmod -R o+rx /path/to/folder_containing_jar_fileFinally, if you just want to check the permissions use the command
ls -altr
and check the final 3 values in the permissions output.
I get a org.datanucleus.exceptions.NucleusUserException: Please check your CLASSPATH and plugin specification error when attempting to connect to Hive. How can I fix that?
Make sure
hive-site.xml
is configured in/etc/hive/conf
and not in/etc/hadoop/conf
.
I get a “Permission Denied” error during Hive import. How do I fix this?
If you see the following error, your Driverless AI instance may not be able to create a temporary Hive folder due to file system permissions restrictions.
ERROR HiveAgent: Error during execution of query: java.lang.RuntimeException: java.lang.RuntimeException: java.io.IOException: Permission denied; org.apache.spark.sql.AnalysisException: java.lang.RuntimeException: java.lang.RuntimeException: java.io.IOException: Permission denied;To fix this error, add the following name-value pair to your
hive-site.xml
file to specify the location that is accessible to Driverless AI (that is, your Driverless AI/tmp
directory).<property> <name>hive.exec.local.scratchdir</name> <value>/path/to/dai/tmp</value> </property>
Recipes
Where can I retrieve H2O’s custom recipes?
H2O’s custom recipes can be obtained from the official Recipes for Driverless AI repository.
How can I create my own custom recipe?
Refer to the How to Write a Recipe guide for details on how to create your own custom recipe.
Are MOJOs supported for experiments that use custom recipes?
In most cases, MOJOs will not be available for custom recipes. Unless the recipe is simple, creating the MOJO is only possible with additional MOJO runtime support. Contact support@h2o.ai for more information about creating MOJOs for custom recipes. (Note: The Python Scoring Pipeline features full support for custom recipes.)
How can I use BYOR in my airgapped installation?
If your Driverless AI environment cannot access Internet and, thus, cannot access Driverless AI’s “Bring Your Own Recipes” from GitHub, please contact H2O support. We can work with you directly to help you access recipes.
When enabling recipes in Driverless AI, can I install Python packages from my organization’s internal Python package index?
Yes—you can use the
pip_install_options
TOML option to specify your organization’s internal Python package index as follows:pip_install_options="['--extra-index-url', 'http://my-own-repo:port']"For more information on the
--extra-index-url <url>
pip install option, refer to the official pip documentation.
Experiments
How much memory does Driverless AI require in order to run experiments?
Right now, Driverless AI requires approximately 10x the size of the data in system memory.
How many columns can Driverless AI handle?
Driverless AI has been tested on datasets with 10k columns. When running experiments on wide data, Driverless AI automatically checks if it is running out of memory, and if it is, it reduces the number of features until it can fit in memory. This may lead to a worse model, but Driverless AI shouldn’t crash because the data is wide.
How should I use Driverless AI if I have large data?
Driverless AI can handle large datasets out of the box. For very large datasets (more than 10 billion rows x columns), we recommend sampling your data for Driverless AI. Keep in mind that the goal of driverless AI is to go through many features and models to find the best modeling pipeline, and not to just train a few models on the raw data (H2O-3 is ideally suited for that case).
For large datasets, the recommended steps are:
Run with the recommended accuracy/time/interpretability settings first, especially accuracy <= 7
Gradually increase accuracy settings to 7 and choose accuracy 9 or 10 only after observing runs with <= 7.
How does Driverless AI detect the ID column?
The ID column logic is one of the following:
The column is named ‘id’, ‘Id’, ‘ID’ or ‘iD’ exactly
The column contains a significant number of unique values (above
max_relative_cardinality
in the config.toml file or Max. allowed fraction of uniques for integer and categorical cols in Expert settings)
Can Driverless AI handle data with missing values/nulls?
Yes, data that is imported into Driverless AI can include missing values. Feature engineering is fully aware of missing values, and missing values are treated as information - either as a special categorical level or as a special number. So for target encoding, for example, rows with a certain missing feature will belong to the same group. For Categorical Encoding where aggregations of a numeric columns are calculated for a grouped categorical column, missing values are kept. The formula for calculating the mean is the sum of non-missing values divided by the count of all non-missing values. For clustering, we impute missing values. And for frequency encoding, we count the number of rows that have a certain missing feature.
The imputation strategy is as follows:
XGBoost/LightGBM do not need missing value imputation and may, in fact, perform worse with any specific other strategy unless the user has a strong understanding of the data.
Driverless AI automatically imputes missing values using the mean for GLM.
Driverless AI provides an imputation setting for TensorFlow in the config.toml file:
tf_nan_impute_value post-normalization
. If you set this option to 0, then missing values will be imputed. Setting it to (for example) +5 will specify 5 standard deviations outside the distribution. The default for TensorFlow is -5, which specifies that TensorFlow will treat NAs like a missing value. We recommend that you specify 0 if the mean is better.More information is available in the Missing and Unseen Values Handling section.
How does Driverless AI deal with categorical variables? What if an integer column should really be treated as categorical?
If a column has string values, then Driverless AI will treat it as a categorical feature. There are multiple methods for how Driverless AI converts the categorical variables to numeric. These include:
One Hot Encoding: creating dummy variables for each value
Frequency Encoding: replace category with how frequently it is seen in the data
Target Encoding: replace category with the average target value (additional steps included to prevent overfitting)
Weight of Evidence: calculate weight of evidence for each category (http://ucanalytics.com/blogs/information-value-and-weight-of-evidencebanking-case/)
Driverless AI will try multiple methods for representing the column and determine which representation(s) are best.
If the column has integers, Driverless AI will try treating the column as a categorical column and numeric column. It will treat any integer column as both categorical and numeric if the number of unique values is less than 50.
This is configurable in the config.toml file:
# Whether to treat some numerical features as categorical # For instance, sometimes an integer column may not represent a numerical feature but # represents different numerical codes instead. num_as_cat = true # Max number of unique values for integer/real columns to be treated as categoricals (test applies to first statistical_threshold_data_size_small rows only) max_int_as_cat_uniques = 50(Note: Driverless AI will also check if the distribution of any numeric column differs significantly from the distribution of typical numerical data using Benford’s Law. If the column distribution does not obey Benford’s Law, we will also try to treat it as categorical even if there are more than 50 unique values.)
How are outliers handled?
Outliers are not removed from the data. Instead Driverless AI finds the best way to represent data with outliers. For example, Driverless AI may find that binning a variable with outliers improves performance.
For target columns, Driverless AI first determines the best representation of the column. It may find that for a target column with outliers, it is best to predict the log of the column.
If I drop several columns from the Train dataset, will Driverless AI understand that it needs to drop the same columns from the Test dataset?
If you drop columns from the training dataset, Driverless AI will do the same for the validation and test datasets (if the columns are present). There is no need for these columns because no features will be created from them.
Does Driverless AI treat numeric variables as categorical variables?
In certain cases, yes. You can prevent this behavior by setting the
num_as_cat
variable in your installation’s config.toml file tofalse
. You can have finer grain control over this behavior by excluding theNumeric to Categorical Target Encoding Transformer
and theNumeric To Categorical Weight of Evidence Transformer
and their corresponding genes in your installation’s config.toml file. To learn more about the config.toml file, see the Using the config.toml File section.
Which algorithms are used in Driverless AI?
Features are engineered with a proprietary stack of Kaggle-winning statistical approaches including some of the most sophisticated target encoding and likelihood estimates based on groupings, aggregations and joins, but we also employ linear models, neural nets, clustering and dimensionality reduction models and many traditional approaches such as one-hot encoding etc.
On top of the engineered features, sophisticated models are fitted, including, but not limited to: XGBoost (both original XGBoost and ‘lossguide’ (LightGBM) mode), Decision Trees, GLM, TensorFlow (including a TensorFlow NLP recipe based on CNN Deeplearning models), RuleFit, FTRL (Follow the Regularized Leader), Isolation Forest, and Constant Models. (Refer to Supported Algorithms for more information.) And additional algorithms can be added via Recipes.
In general, GBMs are the best single-shot algorithms. Since 2006, boosting methods have proven to be the most accurate for noisy predictive modeling tasks outside of pattern recognition in images and sound (https://www.cs.cornell.edu/~caruana/ctp/ct.papers/caruana.icml06.pdf). The advent of XGBoost and Kaggle only cemented this position.
Why do my selected algorithms not show up in the Experiment Preview?
When changing the algorithms used via Expert Settings > Model and Expert Settings > Recipes, you may notice in the Experiment Preview that those changes are not applied. Driverless AI determines whether to include models and/or recipes based on a hierarchy of those expert settings as well as data types (numeric, categorical, text, image, etc.) and system properties (GPUs, multiple GPUs, etc.).
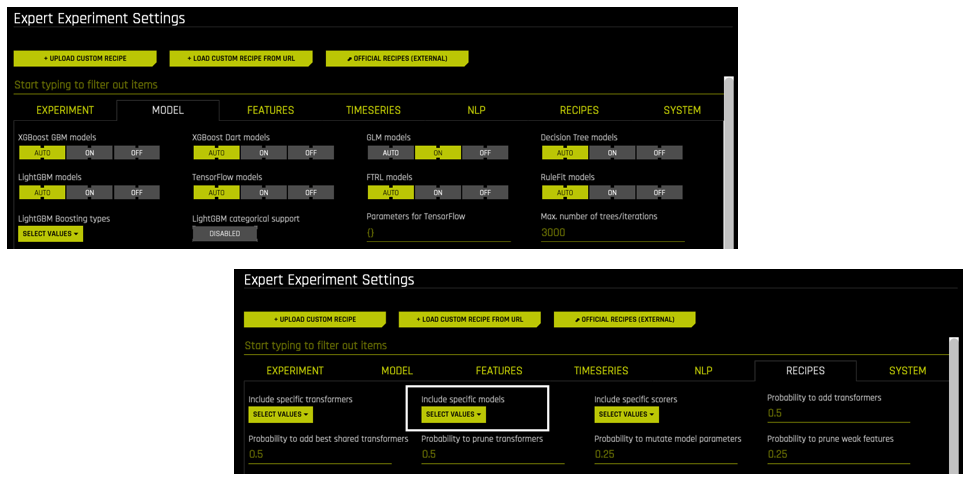
Setting an Algorithm to “OFF” in Expert Settings: If an algorithm is turned OFF in Expert Settings (for example, GLM Models) when running, then that algorithm will not be included in the experiment.
Algorithms Not Included from Recipes (BYOR): If an algorithm from a custom recipe is not selected for the experiment in the Include specific models option, then that algorithm will not be included in the experiment, regardless of whether that same algorithm is set to AUTO or ON on the Expert Settings > Model page.
Algorithms Not Specified as “OFF” and Included from Recipes: If a Driverless AI algorithm is specified as either “AUTO” or “ON” and additional models are selected for the experiment in the Include specific models option, than those algorithms may or may not be included in the experiment. Driverless AI will determine the algorithms to use based on the data and experiment type.
To show warnings in the preview for which models were not used, set
show_inapplicable_models_preview = true
in config.toml
Why do my selected transformers not show up in the Experiment Preview?
When changing the transformers used via Expert Settings > Transformers and Expert Settings > Recipes, you may notice in the Experiment Preview that those changes are not applied. Driverless AI determines whether to include transformers can be used based upon data types (numeric, categorical, text, image, etc.) and system properties (GPUs, multiple GPUs, etc.).
Transformers Not Included from Recipes (BYOR): If a transformer from a custom recipe is not selected for the experiment in the Include specific transformers option, then that transformer will not be included in the experiment.
To show warnings in the preview for which models were not used, set
show_inapplicable_transformers_preview = true
in config.toml
How can we turn on TensorFlow Neural Networks so they are evaluated?
Neural networks are considered by Driverless AI, although they may not be evaluated by default. To ensure that neural networks are tried, you can turn on TensorFlow in the Expert Settings:
Once you have set TensorFlow to ON. You should see the Experiment Preview on the left hand side change and mention that it will evaluate TensorFlow models:
We recommend using TensorFlow neural networks if you have a multinomial use case with more than 5 unique values.
Does Driverless AI standardize the data?
Driverless AI will automatically do variable standardization for certain algorithms. For example, with Linear Models and Neural Networks, the data is automatically standardized. For decision tree algorithms, however, we do not perform standardization because these algorithms do not benefit from standardization.
What objective function is used in XGBoost?
The objective function used in XGBoost is:
reg:squarederror
and a custom absolute error objective function for regression
binary:logistic
ormulti:softprob
for classificationThe objective function does not change depending on the scorer chosen. The scorer influences parameter tuning only. For regression, Tweedie, Gamma, and Poisson regression objectives are supported.
More information on the XGBoost instantiations can be found in the logs and in the model summary, both of which can be downloaded from the GUI or found in the /tmp/h2oai_experiment_<name>/ folder on the server.
Does Driverless AI perform internal or external validation?
Driverless AI does internal validation when only training data is provided. It does external validation when training and validation data are provided. In either scenario, the validation data is used for all parameter tuning (models and features), not just for feature selection. Parameter tuning includes target transformation, model selection, feature engineering, feature selection, stacking, etc.
Specifically:
Internal validation (only training data given):
Ideal when data is either close to i.i.d., or for time-series problems
Internal holdouts are used for parameter tuning, with temporal causality for time-series problems
Will do the full spectrum from single holdout split to 5-fold CV, depending on accuracy settings
No need to split training data manually
Final models are trained using CV on the training data
External validation (training + validation data given):
Ideal when there’s some amount of drift in the data, and the validation set mimics the test set data better than the training data
No training data wasted during training because training data not used for parameter tuning
Validation data is used only for parameter tuning, and is not part of training data
No CV possible because we explicitly do not want to overfit on the training data
Not allowed for time-series problems (see Time Series FAQ section that follows)
Tip: If you want both training and validation data to be used for parameter tuning (the training process), just concatenate the datasets together and turn them both into training data for the “internal validation” method.
How does Driverless AI prevent overfitting?
Driverless AI performs a number of checks to prevent overfitting. For example, during certain transformations, Driverless AI calculates the average on out-of-fold data using cross validation. Driverless AI also performs early stopping for every model built, ensuring that the model build will stop when it ceases to improve on holdout data. And additional steps to prevent overfitting include checking for i.i.d. and avoiding leakage during feature engineering.
A blog post describing Driverless AI overfitting protection in greater detail is available here: https://www.h2o.ai/blog/driverless-ai-prevents-overfitting-leakage/.
More aggressive overfit protection can be enabled by setting
lock_ga_to_final_trees=true
to true or usingrecipe='more_overfit_protection'
andfixed_only_first_fold_model='true'
and for time-series experimentsallow_stabilize_varimp_for_ts=true
.
How does Driverless AI avoid the multiple hypothesis (MH) problem?
Driverless AI uses a variant of the reusable holdout technique to address the multiple hypothesis problem. Refer to https://pdfs.semanticscholar.org/25fe/96591144f4af3d8f8f79c95b37f415e5bb75.pdf for more information.
How does Driverless AI suggest the experiment settings?
When you run an experiment on a dataset, the experiment settings (Accuracy, Time, and Interpretability) are automatically suggested by Driverless AI. For example, Driverless AI may suggest the parameters Accuracy = 7, Time = 3, Interpretability = 6, based on your data.
Driverless AI will automatically suggest experiment settings based on the number of columns and number of rows in your dataset. The settings are suggested to ensure best handling when the data is small. If the data is small, Driverless AI will suggest the settings that prevent overfitting and ensure the full dataset is utilized.
If the number of rows and number of columns are each below a certain threshold, then:
Accuracy will be increased up to 8.
The accuracy is increased so that cross validation is done. (We don’t want to “throw away” any data for internal validation purposes.)
Interpretability will be increased up to 8.
The higher the interpretability setting, the smaller the number of features in the final model.
More complex features are not allowed.
This prevents overfitting.
Time will be decreased down to 2.
There will be fewer feature engineering iterations to prevent overfitting.
What happens when I set Interpretability and Accuracy to the same number?
The answer is currently that interpretability controls which features are created and what features are kept. (Also above interpretability = 6, monotonicity constraints are used in XGBoost GBM, XGBoost Dart, LightGBM, and Decision Tree models.) The accuracy refers to how hard Driverless AI then tries to make those features into the most accurate model
Can I specify the number of GPUs to use when running Driverless AI?
When running an experiment, the Expert Settings let you specify the starting GPU ID for Driverless AI to use. You can also specify the maximum number of GPUs to use per model and per experiment. Refer to the Expert Settings section for more information.
How can I create the simplest model in Driverless AI?
To create the simplest model in Driverless AI, set the following Experiment Settings:
Set Accuracy to 1. Note that this can hurt performance as a sample will be used. If necessary, adjust the knob until the preview shows no sampling.
Set Time to 1.
Set Interpretability to 10.
Next, configure the following Expert Settings:
Turn OFF all algorithms except GLM.
Set GLM models to ON.
Set Ensemble level to 0.
Set Select target transformation of the target for regression problems to Identity.
Disable Data distribution shift detection.
Disable Target Encoding.
Alternatively, you can set Pipeline Building Recipe to Compliant. Compliant automatically configures the following experiment and expert settings:
interpretability=10 (To avoid complexity. This overrides GUI or Python client settings for Interpretability.)
enable_glm=’on’ (Remaing algos are ‘off’, to avoid complexity and be compatible with algorithms supported by MLI.)
num_as_cat=true: Treat some numerical features as categorical. For instance, sometimes an integer column may not represent a numerical feature but represent different numerical codes instead.
fixed_ensemble_level=0: Don’t use any ensemble (to avoid complexity).
feature_brain_level=0: No feature brain used (to ensure every restart is identical).
max_feature_interaction_depth=1: Interaction depth is set to 1 (no multi-feature interactions to avoid complexity).
target_transformer=”identity”: For regression (to avoid complexity).
check_distribution_shift=”off”: Don’t use distribution shift between train, valid, and test to drop features (bit risky without fine-tuning).
For information on why your experiment isn’t performing as expected, see experiment_performance.
When I run multiple experiments with different seeds, why do I see different scores, runtimes, and sizes on disk in the Experiments listing page?
When running multiple experiments with all of the same settings except the seed, understand that a feature brain level > 0 can lead to variations in models, features, timing, and sizes on disk. (The default value is 2.) These variations can be disabled by setting the Feature Brain Level to 0 in the Expert Settings or in the config.toml file.
In addition, if you use a different seed for each experiment, then each experiment can be different due to the randomness in the genetic algorithm that searches for the best features and model parameters. Only if Reproducible is set with the same seed and with a feature brain level of 0 should users expect the same outcome. Once a different seed is set, the models, features, timing, and sizes on disk can all vary within the constraints set by the choices made for the experiment. (I.e., accuracy, time, interpretability, expert settings, etc., all constrain the outcome, and then a different seed can change things within those constraints.)
Why does the final model performance appear to be worse than previous iterations?
There are a few things to remember:
Driverless AI creates a best effort estimate of the generalization performance of the best modeling pipeline found so far.
The performance estimation is always based on holdout data (data unseen by the model).
If no validation dataset is provided, the training data is split internally to create internal validation holdout data (once or multiple times or cross-validation, depending on the accuracy settings).
If no validation dataset is provided, for accuracy <= 7, a single holdout split is used, and a “lucky” or “unlucky” split can bias estimates for small datasets or datasets with high variance.
If a validation dataset is provided, then all performance estimates are solely based on the entire validation dataset (independent of accuracy settings).
All scores reported are based on bootstrapped-based statistical methods and come with error bars that represent a range of estimate uncertainty.
After the final iteration, a best final model is trained on a final set of engineered features. Depending on accuracy settings, a more accurate estimation of generalization performance may be done using cross-validation. Also, the final model may be a stacked ensemble consisting of multiple base models, which generally leads to better performance. Consequently, in rare cases, the difference in performance estimation method can lead to the final model’s estimated performance seeming poorer than those from previous iterations. (i.e., The final model’s estimated score is significantly worse than the last iteration score and error bars don’t overlap.) In that case, it is very likely that the final model performance estimation is more accurate, and the prior estimates were biased due to a “lucky” split. To confirm this, you can re-run the experiment multiple times (without setting the reproducible flag).
If you would like to minimize the likelihood of the final model performance appearing worse than previous iterations, here are some recommendations:
Increase accuracy settings
Provide a validation dataset
Provide more data
How can I find features that may be causing data leakages in my Driverless AI model?
To find original features that are causing leakage, have a look at features_orig.txt in the experiment summary download. Features causing leakage will have high importance there. To get a hint at derived features that might be causing leakage, create a new experiment with dials set to 2/2/8, and run the new experiment on your data with all your features and response. Then analyze the top 1-2 features in the model variable importance. They are likely the main contributors to data leakage if it is occurring.
How can I see the performance metrics on the test data?
As long as you provide a target column in the test set, Driverless AI will show the best estimate of the final model’s performance on the test set at the end of the experiment. The test set is never used to tune parameters (unlike to what Kagglers often do), so this is purely a convenience. Of course, you can still make test set predictions and compute your own metrics using a method of your choice.
How can I see all the performance metrics possible for my experiment?
At the end of the experiment, the model’s estimated performance on all provided datasets with a target column is printed in the experiment logs. For example, for the test set:
Final scores on test (external holdout) +/- stddev: GINI = 0.87794 +/- 0.035305 (more is better) MCC = 0.71124 +/- 0.043232 (more is better) F05 = 0.79175 +/- 0.04209 (more is better) F1 = 0.75823 +/- 0.038675 (more is better) F2 = 0.82752 +/- 0.03604 (more is better) ACCURACY = 0.91513 +/- 0.011975 (more is better) LOGLOSS = 0.28429 +/- 0.016682 (less is better) AUCPR = 0.79074 +/- 0.046223 (more is better) optimized: AUC = 0.93386 +/- 0.018856 (more is better)
What if my training/validation and testing data sets come from different distributions?
In general, Driverless AI uses training data to engineer features and train models and validation data to tune all parameters. If no external validation data is given, the training data is used to create internal holdouts. The way holdouts are created internally depends on whether there is a strong time dependence, see the point below. If the data has no obvious time dependency (e.g., if there is no time column neither implicit or explicit), or if the data can be sorted arbitrarily and it won’t affect the outcome (e.g., Iris data, predicting flower species from measurements), and if the test dataset is different (e.g., new flowers or only large flowers), then the model performance on validation (either internal or external) as measured during training won’t be achieved during final testing due to the obvious inability of the model to generalize.
Does Driverless AI handle weighted data?
Yes. You can optionally provide an extra weight column in your training (and validation) data with non-negative observation weights. This can be useful to implement domain-specific effects such as exponential weighting in time or class weights. All of our algorithms and metrics in Driverless AI support observation weights, but note that estimated likelihoods can be skewed as a consequence.
How does Driverless AI handle fold assignments for weighted data?
Currently, Driverless AI does not take the weights into account during fold creation, but you can provide a fold column to enforce your own grouping, i.e., to keep rows that belong to the same group together (either in train or valid). The fold column has to be a categorical column (integers ok) that assigns a group ID to each row. (It needs to have at least 5 groups because we do up to 5-fold CV.)
Why do I see that adding new features to a dataset deteriorates the performance of the model?
You may notice that after adding one or more new features to a dataset, it deteriorates the performance of the Driverless AI model. In Driverless AI, the feature engineering sequence is fairly random and may end up not doing same things with original features if you restart entirely fresh with new columns.
Beginning in Driverless AI v1.4.0, you now have the option to Restart from Last Checkpoint. This lets you pull in a new dataset with more columns, and Driverless AI will more iteratively take advantage of the new columns.
How does Driverless AI handle imbalanced data for binary classification experiments?
If you have data that is imbalanced, a binary imbalanced model can help to improve scoring with a variety of imbalanced sampling methods. An imbalanced model is able to take advantage of most (or even all) of the imbalanced dataset’s positive values during sampling, while a regular model significantly limits the population of positive values. Imbalanced models, however, take more time to make predictions, and they are not always more accurate than regular models. We still recommend that you try using an imbalanced model if your data is imbalanced to see if scoring is improved over a regular model. Note that this information only applies to binary models.
How is feature importance calculated in Driverless AI?
For most models, such as XGBoost or LightGBM models, Driverless AI uses normalized information gain to calculate feature importance. Other estimates of importance are sometimes used for certain models.
I want to have only one LightGBM model in the final pipeline. How can I do this?
You can do this by using Ensemble Levels. To change the ensemble level, use the Ensemble Level for Final Modeling Pipeline expert setting (
fixed_ensemble_level
in the config.toml), which is located in the Model tab. If you want a single model, use level 0. If you are okay with using the same model with hyperparameters but trained with multiple cross validation folds, then use level 1.To use only one model type, use the Include Specific Models expert setting, which is located in the Recipes tab.
For more information, see Ensemble Learning in Driverless AI.
Note
Setting
fixed_ensemble_level = 0
returns a single model trained on one hundred percent of the data, not just a single model type with CV.When the Cross-validate Single Final Model expert setting is enabled (default), the single model with
fixed_ensemble_level = 0
has the optimal number of trees because it is tuned with CV. Disabling this setting is not recommended whenfixed_ensemble_level = 0
.
I want to have only one LightGBM model and no FE. How can I do this?
You can do this by additionally limiting the set of allowed transformations to just the OriginalTransformer, which leaves numeric features in their original form and drops all non-numeric features. To include or exclude specific transformers in your Driverless AI environment, use the Include Specific Transformers expert setting (
included_transformers
in the config.toml), which is located in the Recipes tab. You can also set the Feature Engineering Effort expert setting (feature_engineering_effort
in the config.toml) to 0 to achieve the same effect.For more information, see Driverless AI Transformations.
What is fast approximation in Driverless AI?
Fast approximation is available for both regular and Shapley predictions. It is enabled by default for MLI / AutoDoc and turned off by default for other clients. The extent of approximation can be fully configured or turned off with the fast approximation expert settings. Enabling fast approximation can result in a significant speedup for large prediction tasks like the creation of partial dependence plots and other MLI-related tasks.
Note
The following is a list of expert settings that can be used to configure fast approximation.
Regular predictions:
fast-approx-trees
fast-approx-one-fold
fast-approx-one-model
Shapley predictions:
fast-approx-trees-shap
fast-approx-one-fold-shap
fast-approx-one-model-shap
MLI:
When should fast approximation be turned off?
In situations where a more detailed partial dependence plot or interpretation is required, you may want to disable fast approximation.
Why does the confusion matrix sometimes show decimals instead of whole numbers?
Fractional confusion matrix values most commonly arise as a consequence of the averaging of confusion matrices across cross-validation fold splits or across repeated fold splits, but the same can also happen for non-integer observation weights.
Is data sampling for multiclass use cases supported?
Data sampling for multiclass use cases is not currently supported. However, it is possible to approximate the data sampling approach by adding more weight in order to penalize rare classes. You can add weight to an individual observation by using a weight column when setting up your experiment. You can also enable LightGBM multiclass balancing by setting the
enable_lightgbm_multiclass_balancing
configuration setting toon
, which enables automatic class weighting for imbalanced multiclass problems.
Feature Transformations
Where can I get details of the various transformations performed in an experiment?
Download the experiment’s log .zip file from the GUI. This zip file includes summary information, log information, and a gene_summary.txt file with details of the transformations used in the experiment. Specifically, there is a details folder with all subprocess logs.
On the server, the experiment specific files are inside the /tmp/h2oai_experiment_<name>/ folder after the experiment completes, particularly h2oai_experiment_logs_<name>.zip and h2oai_experiment_summary_<name>.zip.
Predictions
How can I download the predictions onto the machine where Driverless AI is running?
When you select Score on Another Dataset, the predictions will automatically be stored on the machine where Driverless AI is running. They will be saved in the following locations (and can be opened again by Driverless AI, both for .csv and .bin):
Training Data Predictions: tmp/h2oai_experiment_<name>/train_preds.csv (also saved as .bin)
Testing Data Predictions: tmp/h2oai_experiment_<name>/test_preds.csv (also saved as .bin)
New Data Predictions: tmp/h2oai_experiment_<name>/automatically_generated_name.csv. Note that the automatically generated name will match the name of the file downloaded to your local computer.
Why are predicted probabilities not available when I run an experiment without ensembling?
When Driverless AI provides pre-computed predictions after completing an experiment, it uses only those parts of the modeling pipeline that were not trained on the particular rows for which the predictions are made. This means that Driverless AI needs holdout data in order to create predictions, such as validation or test sets, where the model is trained on training data only. In the case of ensembles, Driverless AI uses cross-validation to generate holdout folds on the training data, so we are able to provide out-of-fold estimates for every row in the training data and, hence, can also provide training holdout predictions (that will provide a good estimate of generalization performance). In the case of a single model, though, that is trained on 100% of the training data. There is no way to create unbiased estimates for any row in the training data. While DAI uses an internal validation dataset, this is a re-usable holdout, and therefore will not contain holdout predictions for the full training dataset. You need cross-validation in order to get out-of-fold estimates, and then that’s not a single model anymore. If you want to still get predictions for the training data for a single model, then you have to use the scoring API to create predictions on the training set. From the GUI, this can be done using the Score on Another Dataset button for a completed experiment. Note, though, that the results will likely be overly optimistic, too good to be true, and virtually useless.
Deployment
What drives the size of a MOJO?
The size of the MOJO is based on the complexity of the final modeling pipeline (i.e., feature engineering and models). One of the biggest factors is the amount of higher-order interactions between features, especially target encoding and related features, which have to store lookup tables for all possible combinations observed in the training data. You can reduce the amount of these transformations by reducing the value of Max. feature interaction depth and/or Feature engineering effort under Expert Settings, or by increasing the interpretability settings for the experiment. Ensembles also contribute to the final modeling pipeline’s complexity as each model has its own pipeline. Lowering the accuracy settings or setting ensemble level to a lower number. The number of features Max. pipeline features also affects the MOJO size. Text transformers are pretty bulky as well and can add to the MOJO size.
To toggle to a smaller mojo during model building with a single click, see - Reduce mojo size under experiment settings of an experiment.
Are MOJOs thread safe?
Yes, all Driverless AI MOJOs are thread safe.
Running the scoring pipeline for my MOJO is taking several hours. How can I get this to run faster?
When running example.sh, Driverless AI implements a memory setting, which is suitable for most use cases. For very large models, however, it may be necessary to increase the memory limit when running the Java application for data transformation. This can be done using the
-Xmx25g
parameter. For example:java -Xmx25g -Dai.h2o.mojos.runtime.license.file=license.sig -cp mojo2-runtime.jar ai.h2o.mojos.ExecuteMojo pipeline.mojo example.csv
Why have I encountered a “Best Score is not finite” error?
Driverless AI uses 32-bit floats by default. You may encounter this error if your data value exceeds 1E38 or if you are resolving more than 1 part in 10 million. You can resolve this error using one of the following methods:
Enable the Force 64-bit Precision option in the experiment’s Expert Settings.
or
Set
data_precision="float64"
andtransformer_precision="float64"
in config.toml.
Time Series
What if my data has a time dependency?
If you know that your data has a strong time dependency, select a time column before starting the experiment. The time column must be in a Datetime format that can be parsed by pandas, such as “2017-11-06 14:32:21”, “Monday, June 18, 2012” or “Jun 18 2018 14:34:00” etc., or contain only integers.
If you are unsure about the strength of the time dependency, run two experiments: One with time column set to “[OFF]” and one with time column set to “[AUTO]” (or pick a time column yourself).
What is a lag, and why does it help?
A lag is a feature value from a previous point in time. Lags are useful to take advantage of the fact that the current (unknown) target value is often correlated with previous (known) target values. Hence, they can better capture target patterns along the time axis.
Why can’t I specify a validation data set for time-series problems? Why do you look at the test set for time-series problems
The problem with validation vs test in the time series setting is that there is only one valid way to define the split. If a test set is given, its length in time defines the validation split and the validation data has to be part of train. Otherwise the time-series validation won’t be useful.
For instance: Let’s assume we have train = [1,2,3,4,5,6,7,8,9,10] and test = [12,13], where integers define time periods (e.g., weeks). For this example, the most natural train/valid split that mimics the test scenario would be: train = [1,2,3,4,5,6,7] and valid = [9,10], and month 8 is not included in the training set to allow for a gap. Note that we will look at the start time and the duration of the test set only (if provided), and not at the contents of the test data (neither features nor target). If the user provides validation = [8,9,10] instead of test data, then this could lead to inferior validation strategy and worse generalization. Hence, we use the user-given test set only to create the optimal internal train/validation splits. If no test set is provided, the user can provide the length of the test set (in periods), the length of the train/test gap (in periods) and the length of the period itself (in seconds).
Why does the gap between train and test matter? Is it because of creating the lag features on the test set?
Taking the gap into account is necessary in order to avoid too optimistic estimates of the true error and to avoid creating history-based features like lags for the training and validation data (which cannot be created for the test data due to the missing information).
In regards to applying the target lags to different subsets of the time group columns, are you saying Driverless AI perform auto-correlation at “levels” of the time series? For example, consider the Walmart dataset where I have Store and Dept (and my target is Weekly Sales). Are you saying that Driverless AI checks for auto-correlation in Weekly Sales based on just Store, just Dept, and both Store and Dept?
Currently, auto-correlation is only applied on the detected superkey (entire TGC) of the training dataset relation at the very beginning. It’s used to rank potential lag-sizes, with the goal to prune the search space for the GA optimization process, which is responsible for selecting the lag features.
How does Driverless AI detect the time period?
Driverless AI treats each time series as a function with some frequency 1/ns. The actual value is estimated by the median of time deltas across maximal length TGC subgroups. The chosen SI unit minimizes the distance to all available SI units.
What is the logic behind the selectable numbers for forecast horizon length?
The shown forecast horizon options are based on quantiles of valid splits. This is necessary because Driverless AI cannot display all possible options in general.
Assume that in my Walmart dataset, all stores provided data at the week level, but one store provided data at the day level. What would Driverless AI do?
Driverless AI would still assume “weekly data” in this case because the majority of stores are yielding this property. The “daily” store would be resampled to the detected overall frequency.
Assume that in my Walmart dataset, all stores and departments provided data at the weekly level, but one department in a specific store provided weekly sales on a bi-weekly basis (every two weeks). What would Driverless AI do?
That’s similar to having missing data. Due to proper resampling, Driverless AI can handle this without any issues.
Why does the number of weeks that you want to start predicting matter?
That’s an option to provide a train-test gap if there is no test data is available. That is to say, “I don’t have my test data yet, but I know it will have a gap to train of x.”
Are the scoring components of time series sensitive to the order in which new pieces of data arrive? I.e., is each row independent at scoring time, or is there a real-time windowing effect in the scoring pieces?
Each row is independent at scoring time.
What happens if the user, at predict time, gives a row with a time value that is too small or too large?
Internally, “out-of bounds” time values are encoded with special values. The samples will still be scored, but the predictions won’t be trustworthy.
What’s the minimum data size for a time series recipe?
We recommended that you have around 10,000 validation samples in order to get a reliable estimate of the true error. The time series recipe can still be applied for smaller data, but the validation error might be inaccurate.
How long must the training data be compared to the test data?
At a minimum, the training data has to be at least twice as long as the test data along the time axis. However, we recommended that the training data is at least three times as long as the test data.
How does the time series recipe deal with missing values?
Missing values will be converted to a special value, which is different from any non-missing feature value. Explicit imputation techniques won’t be applied.
Can the time information be distributed across multiple columns in the input data (such as [year, day, month]?
Currently Driverless AI requires the data to have the time stamps given in a single column. Driverless AI will create additional time features like [year, day, month] on its own, if they turn out to be useful.
What type of modeling approach does Driverless AI use for time series?
Driverless AI combines the creation of history-based features like lags, moving averages etc. with the modeling techniques, which are also applied for i.i.d. data. The primary model of choice is XGBoost.
What’s the idea behind exponential weighting of moving averages?
Exponential weighting accounts for the possibility that more recent observations are better suited to explain the present than older observations.
Logging
How can I reduce the size of the Audit Logger?
An Audit Logger file is created every day that Driverless AI is in use. The
audit_log_retention_period
config variable lets you specify the number of days, after which the audit.log will be overwritten. This option defaults to 5 days, which means that Driverless AI will maintain Audit Logger files for the last 5 days, and audit.log files older than 5 days are removed and replaced with newer log files. When this option is set to0
, the audit.log file will not be overwritten.